Distributed MicroApps
Fractal Computing™
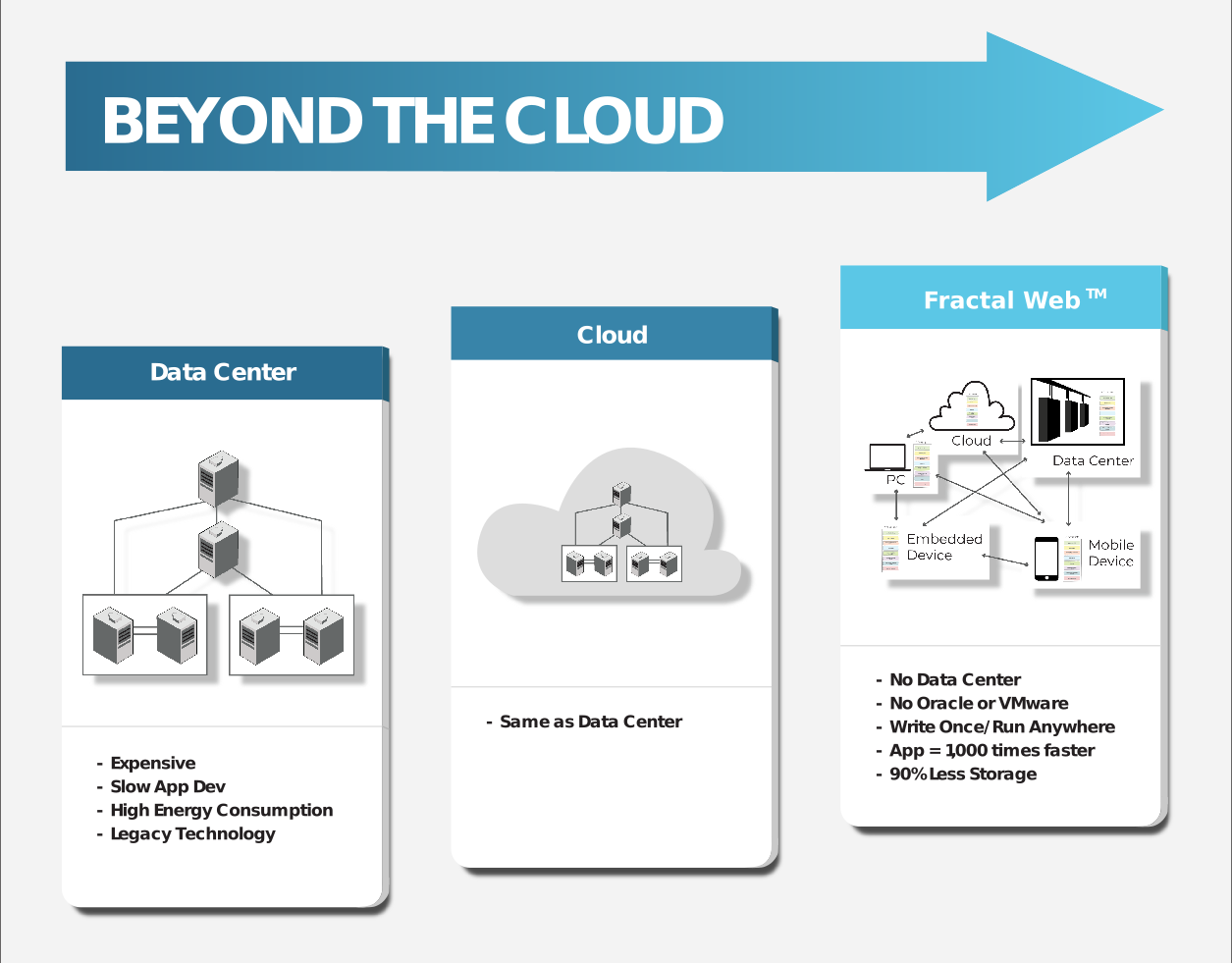
Beyond The Cloud
Distributed MicroApps
History
Initially developed in the early 1980's for a classified government project by a team of MIT & Stanford computer scientists, Fractal Computing required expensive custom hardware.
The technology has matured over 40 years and now runs on inexpensive (Intel NUC class) commodity hardware.
Use Cases
Is best used when price/performance must be improved by orders of magnitude.
Very large data sets, real time ETL, and accelerating AI are excellent use cases.
Fractal Innovation
Wthout Frctal, a computer CPU that is "working" has short burts of activity followed by long periods of waiting (I/O wait states).
The long waiting periods are due to the fact that accessing data from memory, or long term storage devices, is spectacularly slower than processing data in the CPU core.
Fractal Locality Optimization organizes data and constructs software "pipelines" that move data, at the right time, in the rigt sequence, from longer term storage into the CPU core. Data that needs to be accessed together, is kept local to each other ("locality of reference") so that as an application runs, the CPU core accesses data from a cache layer instead of main memory or hard disk.
Fractal Locality Optimization enables orders of magnitude performance increases and a corresponding reduction in hardware requirements for large distributed systems.
Fractal Architecture
AI LLMs (Large Language Models) have been implemented to absorb as much data as possible (ie. everything on the web). Thse "global libraries" are an impressive technical feat, but they miss the mark for real-world business needs.
Business needs are better supported by deeper expertise in specific areas. For example, it's nice that a call center AI agent can quote Shakespeare, but what the business actually needs is simply expertise in the company's products and services.
A Fractal AI architecture enables the construction of collections of "branch libraries" that contain only the knowledge of a specific domain required for specific business needs. These are still non-trivial libraries, but they have orders of magnitude smaller computing requirements and smaller costs.
Fractal Computing™
Architecture Innovation
An ecosystem for enterprise-scale system design, implementation and deployment beyond the cloud.
Fundamental building block Is a "Fractal" which has:
Each Fractal Is a small vertical software stack running in a loosely coupled process that is an independent processing entity in a distributed processing environment. Fractal Computing gives programmers explicit and granular control over sharding and partitioning of the distributed data. Fractals also constructs software data processing/caching pipelines to efficiently move data to the CPU core during run-time processing.
The performance of Fractal Apps™ is due to Locality Optimization™ technology:
Best use cases are enterprise-class applications that support mission critical repetitive business processes. Examples of this are: billing, forecasting, reconcilliation, and other customer care oriented applications.
Empirical results Locality of Reference optimization can reduce I/O wait-states in application layer code by multiple decimal orders of magnitude (compared to a similar legacy application implemented in a relational database). We have customers with production applications that are now running 1,000x to 1,000,000x faster than their legacy application.
Simultaneous innovation Fractal Computing consists of simultaneous innovations in:
Architecture Innovation
An ecosystem for enterprise-scale system design, implementation and deployment.
Fundamental building block is a Fractal. Each Fractal is a small vertical software stack running in a loosely coupled process that is an independent processing entity in a distributed processing environment.
Each Fractal contains the entire application A user, or another application, interacts with a Fractal via web services. The Fractal contains all of the data and code (including all user interface widgets) for the application that the user wishes to run. Each Fractal is a smaller scale version of the overall application.
Billing example if the user is running a billing application, an individual Fractal might be responsible for on the order of about 25,000 customers and all of their associated information. If the user asks the Fractal to "show a list of all customers" the Fractal will respond with a list of 25,000 customers. If the Fractal is running in a Fractal Computing™ ecosystem where it can communicate with all of the other Fractals -- then before the Fractal sends a response back to the user, it broadcasts a message to the other Fractals asking them if they also manage any customers. The Fractal takes all of their responses and folds them into its internal response before sending the final response back to the user. The mechanisms for dissiminating requests out to other Fractals and integrating their results into a core part of Fractal Computing™.
Spreadsheet example If the user wants to build an application with a "spreadsheet" implementation style, each Fractal is responsible for a section of the spreadsheet. Often these type of spreadsheets are organized as multiple "sheets" within the overall spreadsheet. Each Fractal is responsible for it's assigned sheet in the spreadsheet. This Fractal approach to spreadsheet implementation makes is possible to have "infinite" spreadsheets that enable a simple application implementation style (spreadhseet programming) to scale to very large data sets and to utilize massive amounts of parallel processing. Each Fractal manages its iSheet™ sheet in the aggregate spreadsheet in parallel and Fractal Computing provides the programmer with Locality Optimization™ tools to insure parallelism for optimal run-time performance.
Fractal Computing™ peer-to-peer architecture. Each Fractal contains a fully functional application with all of the data and application logic for performing some task. The user experience of interacting with an individual Fractal or the entire network of Fractals is exactly the same -- the result sets are either a fraction of the total data set (when interacting with only a single Fractal) or the entire data set (when interacting with a Fractal that, in turn, is communicating with the other Fractals).
All Fractals are the same A user can attach to any Fractal in the network to initiate a request. Each Fractal is capable of communicating with all of the other Fractals and assembing a complete result for the user. A Fractal Computing architecture enables massive parallel processing, fault-tolerance, and high availability of the overall application since failover and backup logic is also embedded in each Fractal as part of the Fractal Computing ecosystem.
A New Type of App Requires a New Development & Delivery Environment
Minimalist but complete.
A Fractal contains just the necessary essence of the massive software infrastructure resident in the cloud and enterprise scale applications.
Reduce need for data centers A Fractal instance manages storage and compute resources at a granular scale. A Fractal runs on hardware at the network edge or the minimal instance of the cloud. A Fractal runs on mobile devices, network hardware, desktop PCs, departmental workstations, and conventional servers in your data center and in the cloud. If the hardware has a processor, some storage, and a network connection -- it can be a Fractal resource. Fractal enables you to migrate OUT OF DATA CENTERS, reducing your energy footprint.
Distributed processing & storage Fractal is a distributed development and delivery software platform. It enables an enterprise application and its databases to be split into dozens, hundreds, thousands, or millions of loosely coupled distributed processing and storage resources. Decentralized, distributed management of these resources so they behave as a coherent whole is an innate capability of each Fractal instance.
Development community Fractal utilize distributed libraries of features and content for industry specific or use-case specific applications. Business unit and department level resources that understand Javascript programming can contribute to, and make use of, these community libraries to develop and deliver enterprise scale applications in a single business quarter.
Fractal Computing
10 Million Customer Electric Utility Billing App
Example A medium size 10 million customer billing app is typically deployed on 10 Intel NUC's, each running 40 Fractals, for a total of 400 Fractals.
Each Fractal is connected to the other Fractals in a peer-to-peer network. Each Fractal has a complete copy of the application code and is capable of operating completely independently of the other Fractals.
The 40 Fractals on each NUC manage about 25,000 customer each so each NUC handles the processing for about 1 million customers.
Each Fractal Is a small vertical software stack running as a loosely coupled process that is an independent processing entity in a distributed processing network.
Fractal Computing™ gives programmers explicit and granular control over sharding and partitioning of the distributed data.
The Fractal Framework™ constructs software data processing/caching pipelines to efficiently move data from long-term persistent storage, to fast-access storage, to RAM, to layer-2 cache, and to the CPU cores at run-time.
Data sharding & partitioning Each loosely-coupled Fractal typically hosts 3 types of databases.
Time-series "Inputs" (Customer Interactions) | Interaction "Locations" (Service Points) | Time-series "Outputs" (Calculated by App) |
example: Point of Sale, Call Logs, Meter Readings, etc. | example: Location, Account ID's, Customer ID's, etc. | example: Bills, Forecasts, Alerts, etc. |
25,000 customers 100 records/day 10 years of data Total Records = 9 billion per Fractal | 25,000 customers Total Records = 25,000 per Fractal | 25,000 records/month 10 years of data Total Records = 3 million per Fractal |
Databases partitioned by month into 120 partions per shard with 1 shard per Fractal | Databases in 1 partition per shard with 1 shard per Fractal | Databases partitioned by month into 120 partitions per shard with 1 shard per Fractal |
Total Input Records: (9 Billion / Fractal) x 400 Fractals = 3.6 trillion | Total Location records: (25,000 / Fractal) x 400 Fractals = 10 million | Total Output Records: (3 million / Fractal) x 400 Fractals = 1.2 billion |
Data flow Input data is streamed by the service point DB which guides calculations to produce the output data. This processing is done via a software processing pipeline that optimizes data flow so that data is paged into layer-2 cache immediately prior to a CPU core requiring the next data block from a data stream.
A Journey Worth Taking
Real Transformation
Beyond The Cloud